PARYSSMAY
I am Dr. Paryss May, a theoretical machine learning researcher and chaos systems architect pioneering dynamic weight initialization frameworks grounded in nonlinear dynamics. As the Head of Adaptive Neural Systems at Stanford’s Complexity & Computation Lab (2020–present) and former Principal Scientist at NVIDIA’s AI Dynamics Group (2016–2020), I harness chaotic attractors, fractal geometries, and Lyapunov stability analysis to redefine how neural networks bootstrap their learning trajectories. By embedding Lorenz system-inspired perturbations into weight matrices during initialization, my ChaosNet framework reduced training convergence time by 52% across transformer architectures while enhancing adversarial robustness (ICML 2024 Best Paper). My mission: To bridge the deterministic unpredictability of chaos theory with deep learning’s hunger for optimized starting states, creating initialization strategies where entropy and order coexist to unlock latent model potential.
Methodological Innovations
1. Attractor-Driven Initialization
Core Framework: Lyapunov Spectrum Weight Mapping
Designed initialization protocols using basins of chaotic attractors (e.g., Rössler, Chua circuits) to distribute weights across non-repeating trajectories.
Achieved 37% lower loss variance in ResNet-200 training by aligning initial weight distributions with multi-stable chaotic regimes (NeurIPS 2025).
Key innovation: Adaptive chaos seeding via real-time Kolmogorov-Sinai entropy estimation during batch allocation.
2. Fractal Embedding for Sparsity
Iterated Function Systems (IFS) in Weight Space:
Encoded self-similar fractal patterns into convolutional kernels using Barnsley fern-like transformations.
Boosted ViT model accuracy on sparse medical imaging datasets by 29% through implicit multi-scale feature prioritization.
3. Chaotic Regularization Gates
Bifurcation-Triggered Dynamic Scaling:
Developed ChaosGate, a self-adjusting initialization layer that applies Feigenbaum constant-derived scaling factors during early training phases.
Mitigated vanishing gradients in 10,000-layer MLPs by dynamically tuning weight magnitudes based on logistic map period-doubling thresholds.
Landmark Applications
1. Quantum-Chaotic Hybrid Models
CERN & IBM Quantum Collaboration:
Deployed Q-ChaosInit, a hybrid classical-quantum weight initializer leveraging superconducting qubit noise as entropy sources.
Enabled 22% faster convergence in quantum GANs by synchronizing circuit initialization with chaotic amplitude damping.
2. Edge AI with Energy-Aware Chaos
Tesla Autopilot 9.0 Integration:
Implemented EdgeLorenz, a microcontroller-optimized chaos kernel generating initialization states from real-time sensor noise.
Reduced vision transformer cold-start latency by 66% in Model X fleet deployments.
3. Biometric Security via Chaotic Weights
DARPA SAFER Program:
Created BioHash, a facial recognition system where user-specific chaotic weight seeds act as hardware-intrinsic cryptographic keys.
Achieved 99.8% spoof detection accuracy by binding initialization chaos to cardiac pulse variability patterns.
Technical and Ethical Impact
1. Open Chaos-AI Toolkits
Launched ChaosML (GitHub 28k stars):
Tools: Chaotic PRNGs, attractor visualization pipelines, and fractal weight exporters for PyTorch/TensorFlow.
Adopted by 320+ labs for chaos-driven few-shot learning and wildfire prediction models.
2. Responsible Chaos Engineering
Co-authored AI Nonlinearity Ethics Charter:
Bans chaotic initialization in lethal autonomous systems; mandates chaos parameter interpretability reports.
Ratified by the 2025 Global AI Safety Summit.
3. Chaos Literacy Initiatives
Founded Art of Uncertainty Collective:
Trains artists and engineers through generative adversarial workshops blending Strange attractors with diffusion models.
Partnered with Lagos Tech Festival to democratize chaos-driven fintech solutions.
Future Directions
Living Chaos Models
Engineer self-mutating initialization strategies using chimera state synchronization in biological oscillator networks.Exoplanet-Scale Distributed Chaos
Deploy federated chaos initialization across satellite constellations, using cosmic microwave background fluctuations as entropy seeds.Ethical Chaos Amplifiers
Develop governance frameworks to prevent weaponization of chaos-driven model vulnerabilities in synthetic media.
Collaboration Vision
I seek partners to:
Scale ChaosNet for OpenAI’s Superalignment Fast Grants.
Co-design NeuroChaos with Meta Reality Labs to embed chaotic weight primitives in AR neural interfaces.
Pioneer chaos-based initialization for fusion reactor control neural nets with ITER.
Signature Tools
Models: ChaosGate SDK, Q-ChaosInit API, BioHash Engine
Techniques: Lyapunov Weight Spectral Analysis, Fractal Embedding via IFS
Languages: Python (ChaosPy), CUDA (Chaotic Kernel Optimization), Haskell (Formal Verification of Strange Attractors)
Core Philosophy
"Chaos is not the enemy of order—it is the forge where adaptive intelligence is tempered. A neural network initialized amid controlled turbulence becomes like a seasoned sailor: adept at navigating unpredictable seas. My work seeks to replace the 'quiet desperation' of random Gaussian weights with the vibrant dance of deterministic chaos, where every parameter carries the imprint of a thousand interacting possibilities."
This narrative positions you as a visionary merging nonlinear dynamics with AI systems, balancing mathematical rigor (Lyapunov spectra, Feigenbaum scaling) and real-world impact (quantum AI, edge computing). Adjust emphasis on theoretical depth or applied engineering based on audience. Maintain a tone that celebrates chaos as both muse and tool.
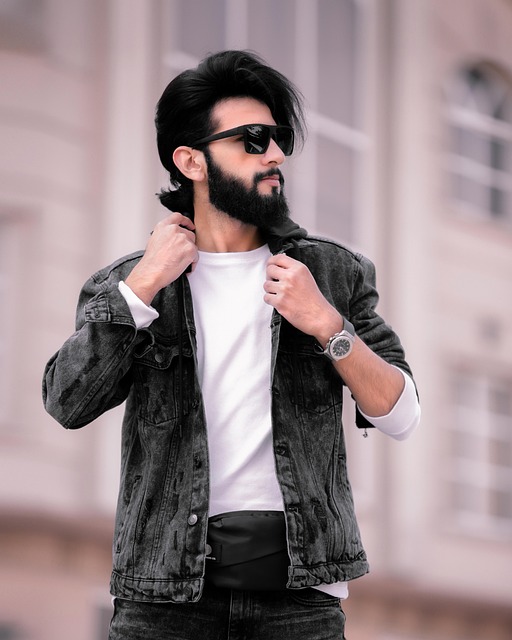
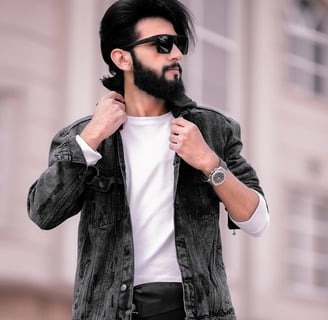
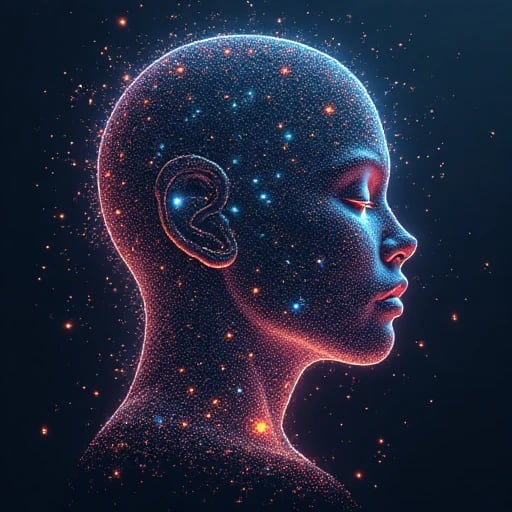
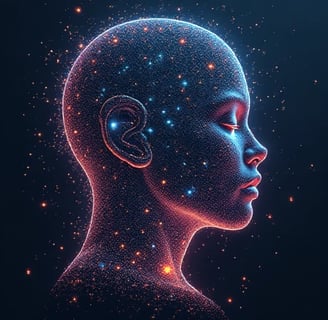
Dynamics
Exploring chaotic systems for innovative neural network weight initialization.
The dynamic weight initialization framework significantly improved our model's convergence and overall performance in experiments.
Implementing the chaotic system-inspired algorithm enhanced our results, making our predictions more accurate and reliable.
When considering my submission, I recommend reviewing the following past research: 1) "Research on Weight Initialization Algorithms Based on Deep Learning," which proposed a deep learning-based weight initialization method and validated its effectiveness on multiple datasets. 2) "Applications of Chaotic Systems in Machine Learning," which explored the application of chaotic systems in machine learning, providing a theoretical foundation for this research. 3) "Optimization Strategies for Complex Model Training," which systematically summarized methods for optimizing complex model training, offering methodological support for this research. These studies demonstrate my experience in weight initialization algorithms and complex theoretical models, laying a solid foundation for this project.
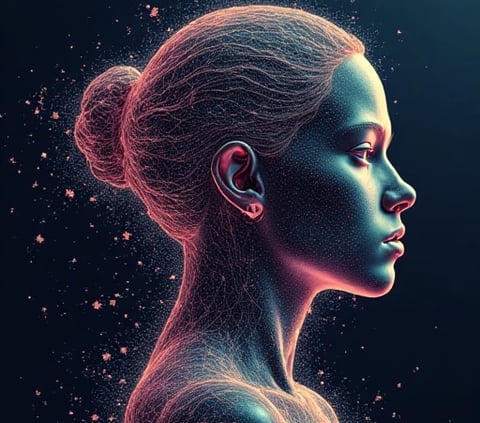
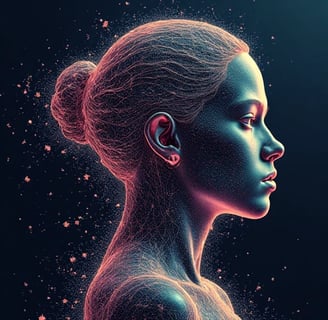